Amazon's Trainium2 AI Accelerator Features 96 GB of HBM, Quadruples Training Performance
by Anton Shilov on November 30, 2023 8:00 AM EST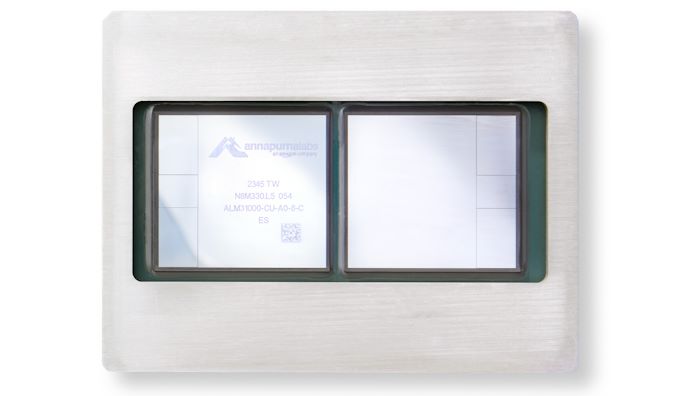
Amazon Web Services this week introduced Trainium2, its new accelerator for artificial intelligence (AI) workload that tangibly increases performance compared to its predecessor, enabling AWS to train foundation models (FMs) and large language models (LLMs) with up to trillions of parameters. In addition, AWS has set itself an ambitious goal to enable its clients to access massive 65 'AI' ExaFLOPS performance for their workloads.
The AWS Trainium2 is Amazon's 2nd Generation accelerator designed specifically for FMs and LLMs training. When compared to its predecessor, the original Trainium, it features four times higher training performance, two times higher performance per watt, and three times as much memory – for a total of 96GB of HBM. The chip designed by Amazon's Annapurna Labs is a multi-tile system-in-package featuring two compute tiles, four HBM memory stacks, and two chiplets whose purpose is undisclosed for now.
Amazon notably does not disclose specific performance numbers of its Trainium2, but it says that its Trn2 instances are scale-out with up to 100,000 Trainium2 chips to get up to 65 ExaFLOPS of low-precision compute performance for AI workloads. Which, working backwards, would put a single Trainium2 accelerator at roughly 650 TFLOPS. 65 EFLOPS is a level set to be achievable only on the highest-performing upcoming AI supercomputers, such as the Jupiter. Such scaling should dramatically reduce the training time for a 300-billion parameter large language model from months to weeks, according to AWS.
Amazon yet has to disclose the full specifications for Trainium2, but we'd be surprised if it didn't add some features on top of what the original Trainium already supports. As a reminder, that co-processor supports FP32, TF32, BF16, FP16, UINT8, and configurable FP8 data formats as well as delivers up to 190 TFLOPS of FP16/BF16 compute performance.
What is perhaps more important than pure performance numbers of a single AWS Trainium2 accelerators is that Amazon has partners, such as Anthropic, that are ready to deploy it.
"We are working closely with AWS to develop our future foundation models using Trainium chips," said Tom Brown, co-founder of Anthropic. "Trainium2 will help us build and train models at a very large scale, and we expect it to be at least 4x faster than first generation Trainium chips for some of our key workloads. Our collaboration with AWS will help organizations of all sizes unlock new possibilities, as they use Anthropic's state-of-the-art AI systems together with AWS’s secure, reliable cloud technology."
Source: AWS
19 Comments
View All Comments
Terry_Craig - Thursday, November 30, 2023 - link
Please refrain from comparing the low-precision performance mentioned by Amazon with the FP64 performance of supercomputers. Thank you :)Kevin G - Thursday, November 30, 2023 - link
I'll second this.Many of this AI operations are lower precision on parse matrices. The TOPs metric (meaning tensor operations per second, not trillions of operations per second) would be a better comparison point as the unit of work is more defined in this context.
Yojimbo - Thursday, November 30, 2023 - link
Where did he do that? He compared the low-precision performance mentioned by Amazon with the low-precision performance of supercomputers. Low-precision performance is what is important here. FP64 performance of Trainium is entirely irrelevant (it doesn't even look like it supports the precision).He did declare that 65 exaflops is of the same level as the 93 exaflops of Jupiter without making it clear that he was comparing 65 with 93. I think that's a stretch.
haplo602 - Friday, December 1, 2023 - link
yeah that is the problem, the linked Jupiter article talks about low precision performance as well, however that is not obvious when reading jut the current text. anyway comparing 65 and 93 is a stretch, that's 50% difference in performance which is a lot ... it's not even in the same ballpark ...Yojimbo - Friday, December 1, 2023 - link
It is obvious they are talking about low precision, as they call Jupiter an "AI supercomputer". Besides, why would one operate under the assumption that the article was making a mistake? The article mentions the low-precision performance of the Trainium 2's and then compares it to Jupiter. So the correct thing would be to compare it to the low-precision performance of Jupiter, which is exactly what it is doing.The problem isn't with the article, the problem is that some people have the idea that FP64 is the "real" way to measure supercomputers, which is bunk. In fact, the majority of new supercomputers aren't on the TOP500 list and their FP64 performance doesn't matter as far as their supercomputing capability. AI is eating HPC. Jupiter itself, which will be on the TOP500, is being built with the idea that AI will be one of its most important workloads.
Dante Verizon - Friday, December 1, 2023 - link
FP64 is a common metric for evaluating supercomputers. While low precision may be acceptable in AI, many fields demand high precision and arithmetic stability, where FP64 is essential.They shouldn't compare projects like Frontier with these focused on AI.
PeachNCream - Thursday, November 30, 2023 - link
I for one welcome our new AI masters and encourage them to cull the weak and the disapproving from among the human population while leaving the rest of us that will not pose a problem to an AI-ruled empire as an interesting and/or amusing science experiment.Oxford Guy - Friday, December 1, 2023 - link
The biggest threat from AI is that it will replace the always-irrational human governance with rational governance — a first.skaurus - Saturday, December 2, 2023 - link
Don't worry, politicians would not allow to replace them until the very last moment possible and then some.easp - Saturday, December 2, 2023 - link
LOL. I'm sure you are much less rational than your self-image.